From loan applications to mobile apps, tailor digital financial services through continuous experimentation.
In financial services, personalization is a key differentiator. Customers demand tailored experiences, whether applying for loans, using banking apps, or engaging with chatbots. Through experimentation and A/B testing, financial institutions can acquire the data to fuel personalized experiences. But experimentation goes beyond personalization—it’s also about maximizing efficiency and reducing guesswork.
By running controlled tests, digital product delivery teams can spend more time building features that customers actually want and less time on ones that flop.
This article explores five ways FinServCo, a fictional financial services company, leverages experimentation to increase engagement, improve business outcomes, and create a more efficient product development process.
Experiment 1: Increasing loan application completion rates
Challenge
FinServCo faced high abandonment rates during the loan application process. The hypothesis was that simplifying the application flow would reduce drop-offs and increase the completion rate by at least 10%.
Experiment approach
Product managers led the redesign of the loan application flow, streamlining the steps and improving clarity. Engineers built and implemented the changes in LaunchDarkly, enabling a controlled rollout of the experiment to a small percentage of users.
Using LaunchDarkly's funnel optimization capabilities, they could precisely track each step in the application process, identifying where users were dropping off and adjusting the flow in real time. Funnel experiments in LaunchDarkly not only helped FinServCo monitor user behavior but also allowed them to experiment with different flows for various user segments. This helped create more efficient paths to conversion.
Lastly, data scientists monitored metrics like completion rates and load times, pulling experiment data securely from LaunchDarkly into their data warehouse for deeper analysis. They used tools like Looker to run additional analyses to, among other things, help correlate user behavior with performance metrics.
Results
The streamlined application process resulted in a 15% increase in loan application completion. The success of this experiment validated that efficient product delivery processes, coupled with data-driven decisions, improve customer engagement.
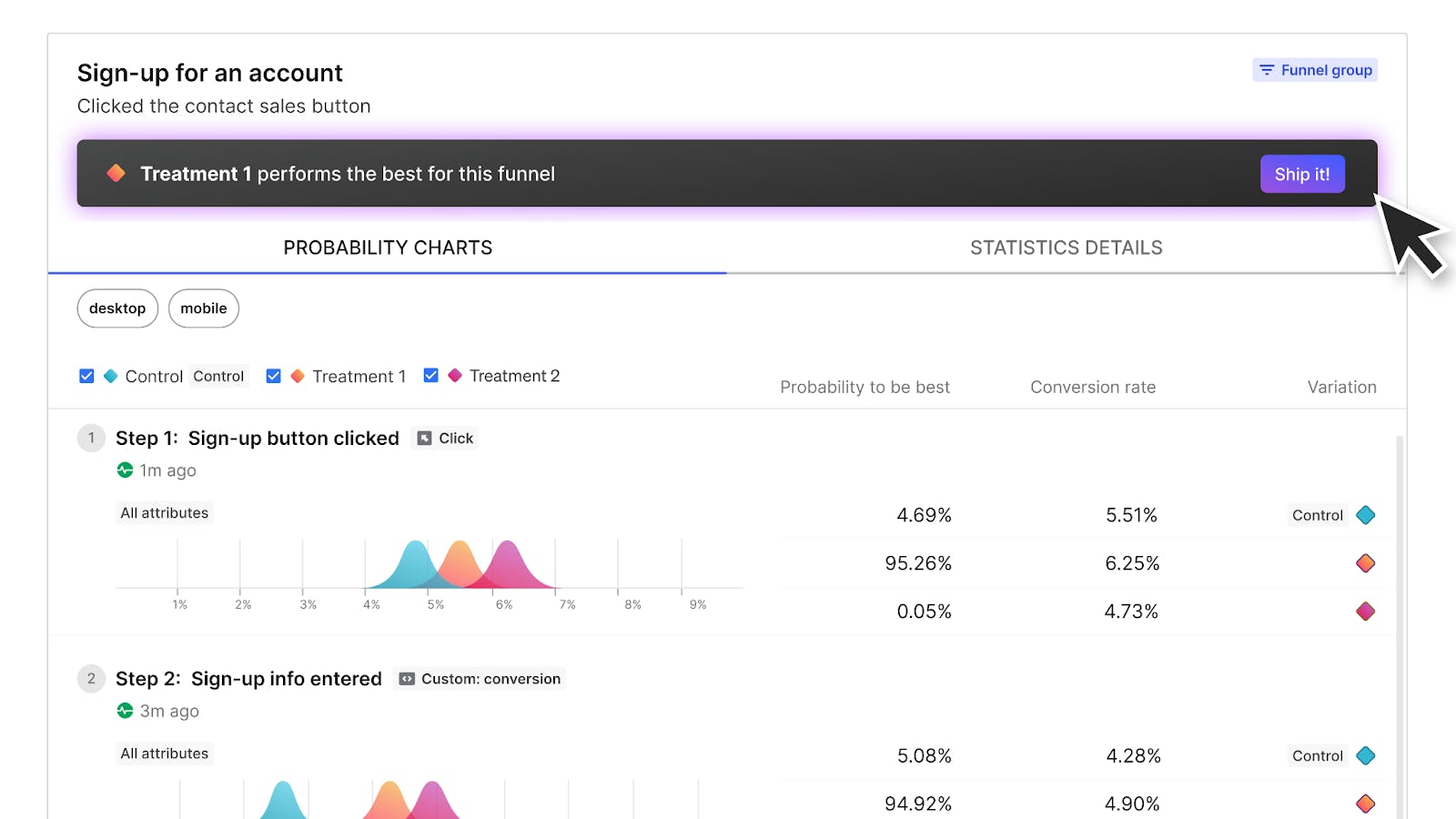
Example: LaunchDarkly funnel optimization experiment
Experiment 2: Improving the mobile banking app experience
Challenge
FinServCo saw declining user engagement with its mobile banking app, particularly when it came to managing accounts. The hypothesis was that a gesture-based navigation would offer a more intuitive experience and improve user engagement.
Experiment approach
Product managers proposed testing two navigation structures: a traditional menu and a gesture-based interface. Engineers implemented both versions using feature flags, enabling them to progressively roll out the experiment, starting with 5% of a targeted segment of highly engaged users. This allowed the team to reduce risk and quickly gather valuable feedback—both qualitative and quantitative.
The product and data team tracked user interaction metrics such as session length and feature usage in their experiment. And they used LaunchDarkly’s results segmentation to explore how the experiment impacted users differently based on what type of mobile device the user was on.
Results
Overall, the gesture-based interface led to a 25% increase in session time and a 10% improvement in account feature usage compared to the traditional menu. This experiment validated the hypothesis, leading to the full rollout of the gesture-based navigation.
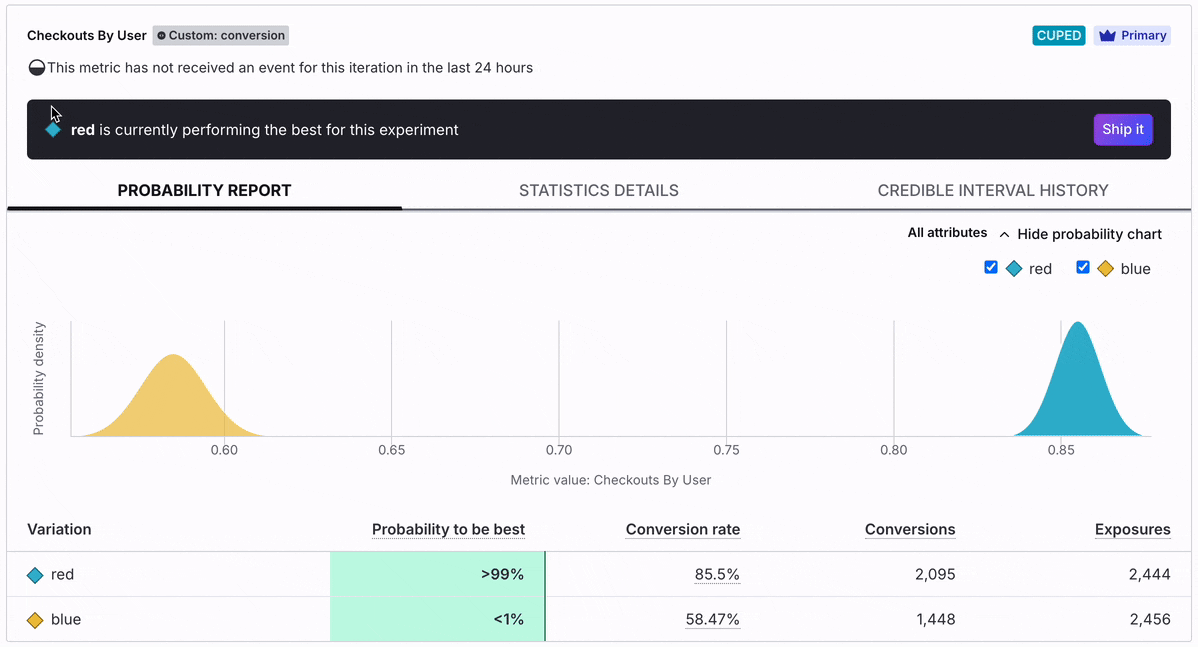
Example: LaunchDarkly feature change experiment
Experiment 3: Enhancing chatbot performance
Challenge
FinServCo’s chatbot was underperforming, with many customers escalating their queries to live agents. Their goal was to improve the chatbot’s resolution rates and reduce escalation. The hypothesis was that a generative AI (GenAI) model would improve the chatbot’s resolution rate by as much as 20%, reducing the need for human intervention.
Experiment approach
The product team worked with engineers to test different chatbot models, comparing scripted responses with a generative AI model. Feature flags allowed a gradual deployment of the AI chatbot experiment, ensuring a controlled environment for the test.
The team also securely exported their experiment data to one of their existing analytics platforms, where data scientists correlated chatbot performance with additional customer satisfaction data.
Results
The generative AI model reduced escalated queries by 30%, leading to an improvement in overall customer satisfaction. The experiment validated that AI-driven responses could significantly enhance chatbot performance, driving both efficiency and customer satisfaction.
Experiment 4: Testing new payment features
Challenge
FinServCo wanted to introduce a “Pay Later” feature, but the team was unsure how different placements and messaging would affect customer adoption. The hypothesis was that a more prominent position in the payment flow would increase adoption by at least 15%.
Experiment approach
Product managers designed an A/B test to evaluate different placements and messaging for the “Pay Later” option in the payment flow. Engineers implemented the changes with feature flags, rolling out the experiment to a small group of users. Data scientists used LaunchDarkly’s experiment results to track adoption rates and engagement metrics.
Results
The “Pay Later” option placed prominently within the payment flow led to a 20% increase in adoption rates, confirming the hypothesis that a “louder” placement would drive a desirable impact on usage.
Experiment 5: Optimizing fraud detection algorithms
Challenge
FinServCo wanted to improve the accuracy of its fraud detection system for credit card transactions. The hypothesis was that the new machine learning-based fraud detection model would increase detection accuracy without significantly slowing down transaction processing times, which could negatively impact user experience.
Experiment approach
The engineering team introduced the new model and deployed it to a subset of transactions using feature flags. They focused on backend performance, monitoring transaction processing times to ensure the new model didn’t introduce latency. They exported experiment data about which transactions were monitored by each fraud detection model into a custom report where engineers analyzed transaction speeds and compared them to the previous model.
Results
The experiment showed that the new fraud detection model improved accuracy without impacting transaction processing times, enabling FinServCo to enhance fraud protection while maintaining system performance.
How LaunchDarkly powers experimentation for financial services
LaunchDarkly enables financial institutions to run safer experiments at scale. Teams can test new front-end features and back-end optimizations with small user segments before fully launching, helping to reduce risk and ensure that only the most effective features get shipped.
By making it easy to connect experiment audience exposure data into existing data tooling infrastructure, LaunchDarkly helps financial services organizations rapidly gain and act upon data-driven insights. This enables greater collaboration among product managers, engineers, and data scientists. In doing so, experimentation not only enhances customer engagement but also increases the efficiency of product development.
Conclusion: Transforming financial services with experimentation
By weaving experimentation into the fabric of their product development processes, financial services organizations can create personalized, efficient, and impactful customer experiences. From loan applications to mobile banking apps, chatbots, and payment systems, experimentation empowers teams to focus on features that matter, reducing wasted effort and improving overall efficiency.
Platforms like LaunchDarkly enable organizations to run safer experiments at scale, driving better business outcomes while maintaining compliance and security.